Modeling accreting black holes
I study accreting supermassive black holes by combining general relativistic magnetohydrodynamical (fluid plasma) simulations with a general relativist ray tracing code (RAPTOR). I am one of the core developers of RAPTOR. I also use this code to generate Virtual Reality visualizations that are used for public outreach and education.
© J.Davelaar 2018
One of my foci is the modeling of the accreting black holes in the centre of our own Milky Way, called Sagittarius A*, and the black hole in centre of the galaxy Messier 87. These two sources are the primary targets of the Event Horizon Telescope.
Additionally, I also study radiation coming from black hole binaries. When the binary is seen edge it produces a self-lensing flare. I discovered that this self-lensing flare can contain a feature that directly correlates with the size of the black hole shadow.
Kinetic plasma simulations
Black holes launch relativistic jets, which are observed over the entire electromagnetic spectrum. The emission shows that the radiative important electron population is partly non-thermal. The way jets accelerated the electron population is part of active debate. During my Ph.D. program, I performed first-principles kinetic plasma simulations of the kink instability. This instability is thought to be important for dissipating magnetic energy and is capable of accelerating electrons to high energies.
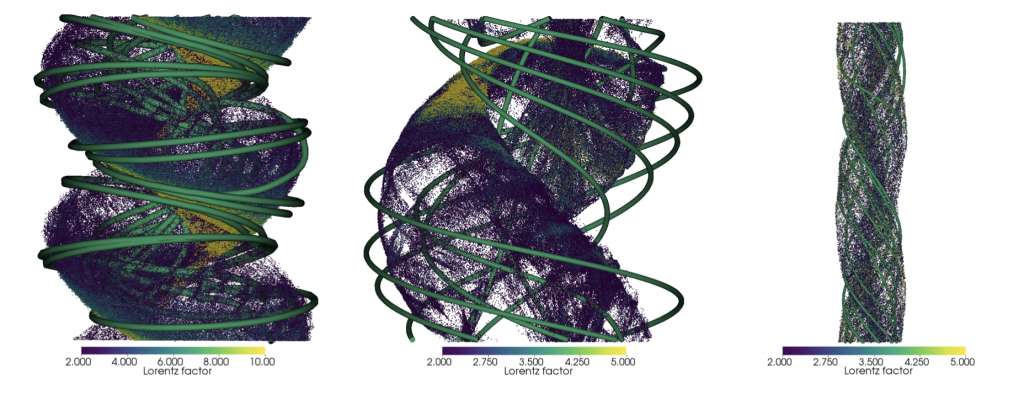
Machine Learning
The extraction of black hole parameters, such as mass, spin, and accretion rate from potential VLBI images of black holes is of vital importance to accurately test our understanding of General Relativity and plasma physics. Convolutional neural networks are known to accurately predict patterns. I am involved in the development of a Bayesian neural network. The network is trained on state-of-the-art simulations of accretion black holes.
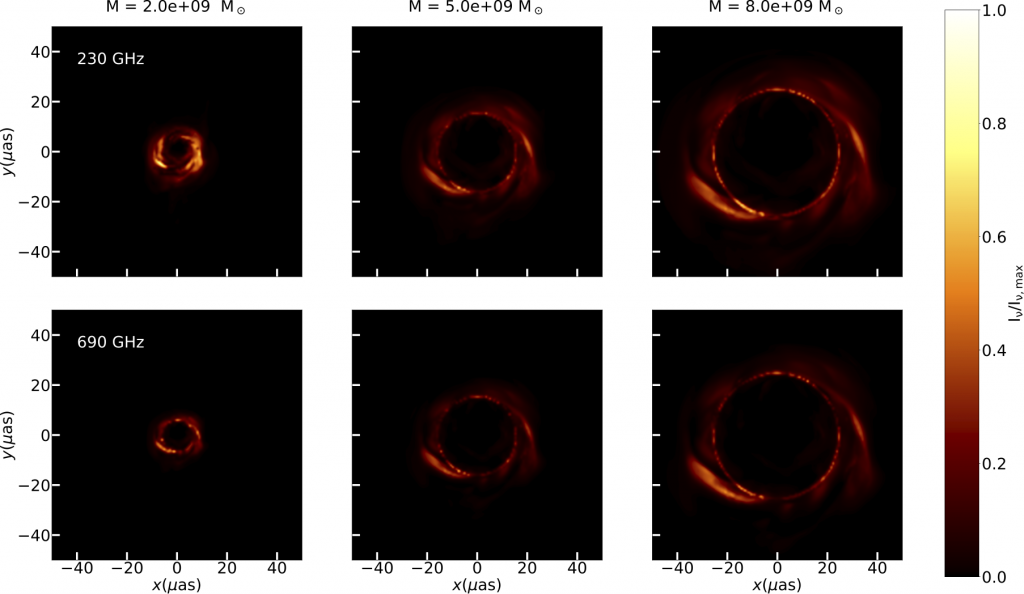